My New Scopus Indexed Paper!
- Alvita Rd
- Nov 15, 2018
- 3 min read
Finally, after a couple of months waiting, I can proudly present my paper published by AIP Proceeding with Scopus Index! *throws confetti*
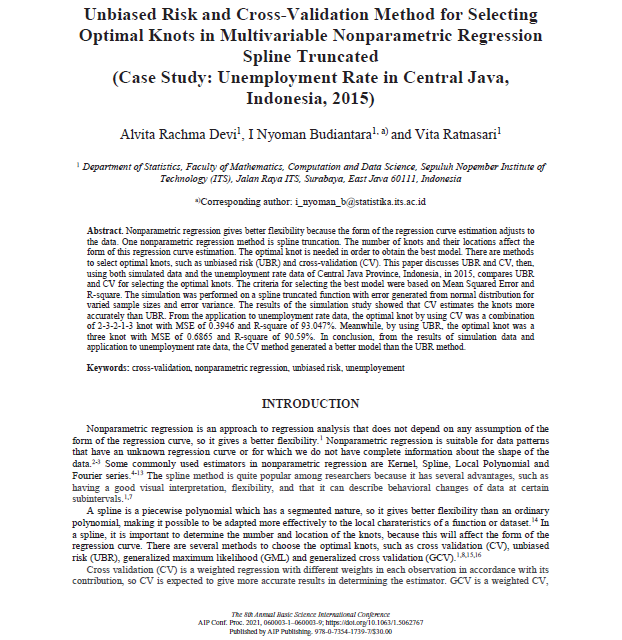
This is my first Scopus indexed paper, and I couldn’t be happier! My research, with my blood-sweat-tears, is appreciated enough to be published in AIP Proceeding. I wish it can be a motivation for me to do more research, write more paper, attend more seminar.
A brief explanation about this paper, this is a comparison between two methods of choosing optimal knot in spline regression. Hmm, I know you may think, “I don’t get a word you said.” Easy peasy! I will walk you through it!
So, if you like research, or maybe just a college student who do assignment that need statistics, ypu must have heard about regression. Simply said, regression explain the relation between two or more variable, called response and predictors. Predictor is a variable which affect the response variable. Let’s say, your expense for a week is influenced by how much pocket-money you get from you mother. If this week you mother get angry with you and not giving you any pocket money, with assumption you have zero money, then you can’t buy anything for this week. So, your expense is response, while your pocket-money or income is predictors.
In regression, there are two kind of approach; parametric and non-parametric. People commonly use parametric method, like linear regression. In parametric regression, there are some assumption that you have to fulfill in order you get the best results. However, in a lot of case, you can not fulfill those assumption. The alternative are; you can fix your data, or you can use nonparametric regression.
Nonparametric regression have a more flexible assumption. Why? Because when you use nonparametric, basically the estimated form of regression curve is expected to adjust to data without being influenced by researcher’s subjectivity (Eubank, 1999). Wow, then why people not just using nonparametric regression?, you may think. Well, because, one, it’s not as simple as parametric regression. Two, it needs high computation.
If your data fit best with parametric regression and met its assumption, then I think it’s unnecessary to use nonparametric regression. Let’s say you are a doctor. One day, you have a patient who have influenza symptoms. Of course you give her/him an influenza medicine. You don’t need to send her/him to the lab or even send her/him to operation room. That’s the analogy of using parametric and nonparametric regression.
In nonparametric regression, there are a lot of estimators, such as Spline, Kernel, Fourier Series, Wavelet, etc. The most commonly used estimator is Spline. In Spline, it is important to determine the number and location of the knots, because this will affect the form of the regression curve. And because in determining the optimal knot is very important, you have to choose it carefully right? There are methods for choosing the optimal knots, such as cross validation (CV), unbiased risk (UBR), generalized maximum likelihood (GML) and generalized cross validation (GCV). And in this paper, I study about CV and UBR method, to understand which method gives the best results. And I study both method by using simulation and application on unemployment rate data.
So, that’s a brief explanation about my paper. Still confuse? Don’t worry, I will explain more about nonparametric regression and Spline on the next post.
If you’re interested on these topics, I recommended you to read Eubank (1999), Wahba (1990) or Wang (2011). Or maybe you can download my paper here or all of my published paper here.
Please contact me if you have any question!
Reading material:
Eubank R L 1999 Nonparametric Regression and Spline Smoothing (New York: Marcel Dekker)
Wahba G 1990 Spline Model for Observational Data (Philadelphia: SIAM)
Wang Y 2011 Smoothing Splines Methods and Applications (California: CRC Press)
Comments